Study: Using satellite images and deep learning to map urban slums in Africa
A new study combines satellite images and deep learning to map slums in Africa’s major cities. It reveals strong slum growth in the urban periphery between 2016 and 2022. The findings allow timely and cost-effective slum monitoring and facilitate targeted interventions.
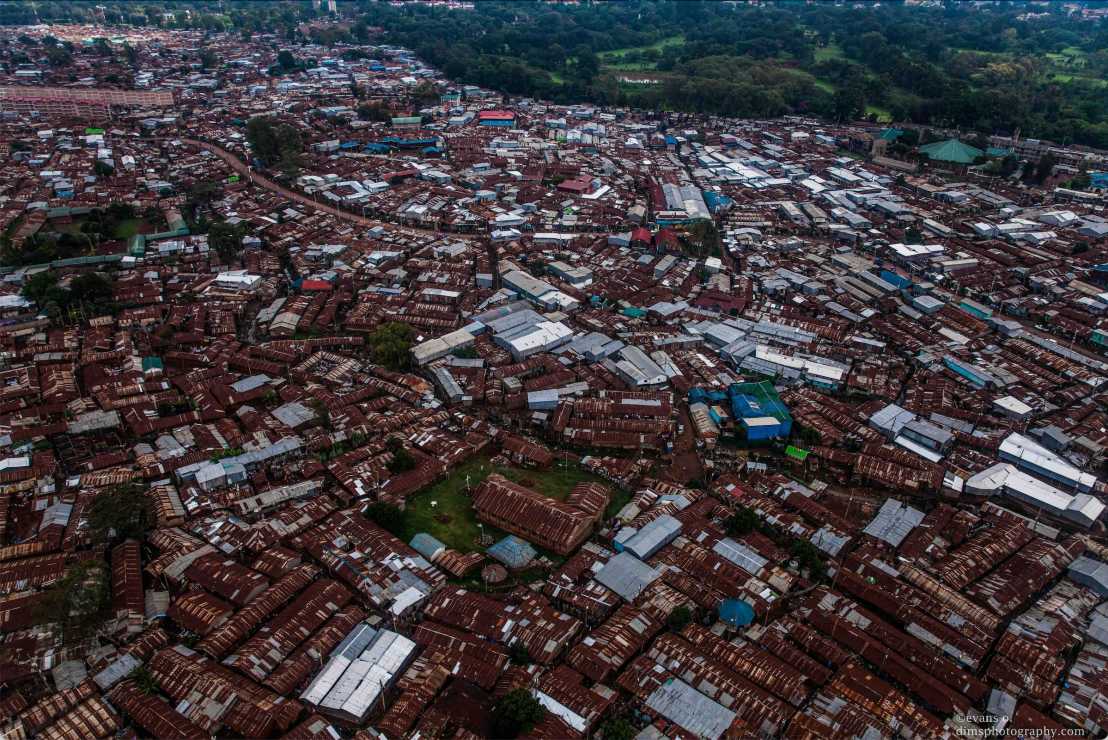
Sub-Saharan Africa is the world’s fastest urbanizing region, and half of its urban residents live in slums – often in unstable and overcrowded dwellings and lacking access to clean water and sanitation. At the same time, the United Nations Sustainable Development Goal (SDG) 11.1. aims to “ensuring access for all to adequate, safe and affordable housing and basic services and upgrade slums” by 2030.
A recent study by Nicolas Büttner from ETH's Development Economics group and NADEL's Kenneth Harttgen, published in Habitat International, combines open-access satellite images and deep learning to map slums in Africa’s major cities and facilitate timely and cost-effective monitoring of SDG 11.1. The approach is based on training a neural network with so-called ground truth data of known slum boundaries. In a train-validate-test setup, the algorithm learns iteratively to detect slums in satellite images – based on morphological features, such as a densely and irregularly built shacks.
The study found that in 2022 the share of slum dwellers exceeded 50% in half of Africa’s major cities, and in most cities this share increased between 2016 and 2022. Slum growth occurred primarily in the urban periphery, which tends to be missed in conventional survey-based slum monitoring.